Vincent Vanhoucke is Google’s chief scientist and Ph.D. in electrical engineering from Stanford University. He currently leads robot-related projects at Google Brain. Vanhoucke's main research areas are speech recognition, computer vision, and robotics. He will also host the Conference on Robot Learning CoRL 2017 (Conference on Robot Learning). Vanhoucke believes that machine intelligence has now reached a considerable level of performance that can rival (and even surpass) humans in certain situations, such as machine vision, machine translation, and speech recognition. Now it is time for these capabilities in the physical world. Play the effect. In his speech today, he mentioned that robotics research is now facing a deep learning innovation. To achieve this, it requires the current machine learning practitioners to jump out of the supervised learning comfort zone and face some thorny problems: The data is scarce How to make the machine realize skills conversion and continuous learning and so on. Vanhoucke also mentioned that this is the only way for artificial intelligence to go from theory to practice. Vanhoucke said that in 2011, speech recognition researchers used neural network technology to reduce speech recognition and the error rate was greatly reduced. This is the largest improvement in the field of speech recognition in more than a decade; in 2016, it almost reached the level of humanity.
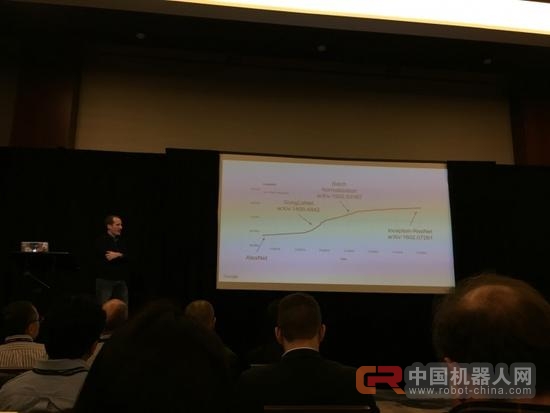
In machine translation, the introduction of machine learning in 2014 also made qualitative progress in machine translation, but it is still relatively untenable to reach human standards...
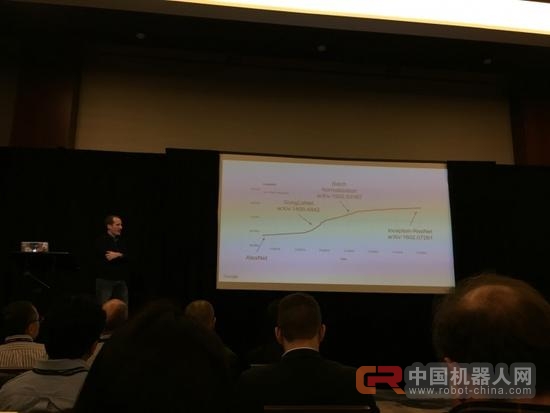
(Looking at the list on the keynote, and contributing to Google's machine translation, there are a lot of Chinese.) The histogram is the difference in the quality of human translation, neural network translation, and PBMT translation.
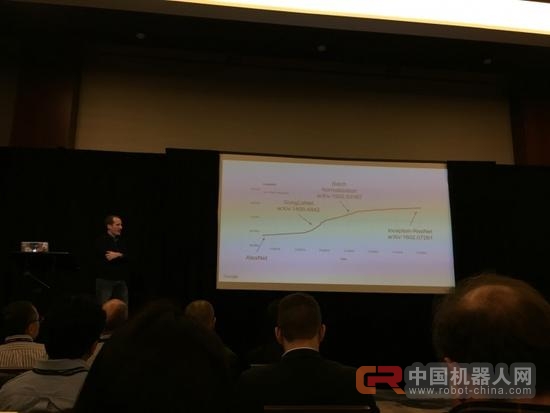
Vanhoucke believes that machine learning research is easier than ever, there are more open source tools and models, more web tutorials (he himself started online courses at Udacity), and the GPU and high-performance computing hardware thresholds have also become lower There are more researchers than ever before.
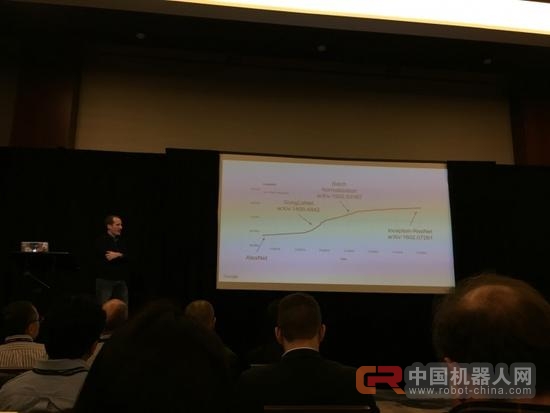
In the field of image recognition, in addition to the improvement of the underlying technology, Google has applied image recognition technology to the medical field to help doctors diagnose the condition and achieved some results.
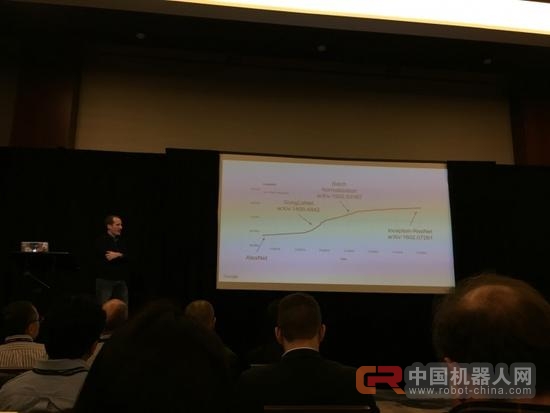
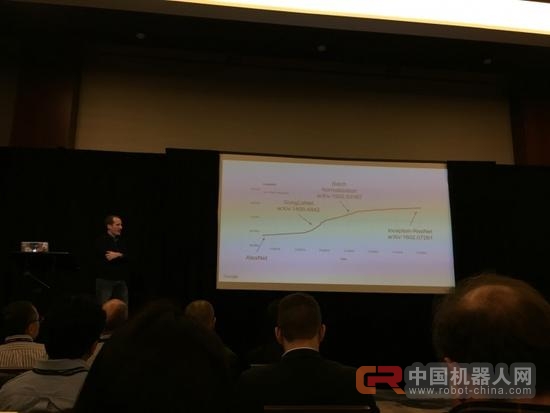
But he also said that image recognition is now far from the point of "succes," and that 40% of decisions based on image monitoring are made. The result is very bad.
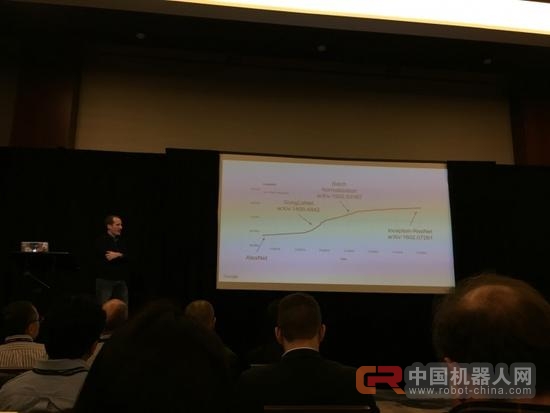
Next is the part of the robot. Vanhoucke is a major in electrical engineering. The main work in Google Brain is robotics. He first stressed the point that a lot of people have different perceptions: Current robotics research has little to do with deep learning. He did a demonstration and dropped the pen on the ground, saying that if the robot's task was to grab the pen, then it caught and couldn't catch it, and there was no difference in the robot's movement from the outside. Therefore, we cannot get any rules from it).
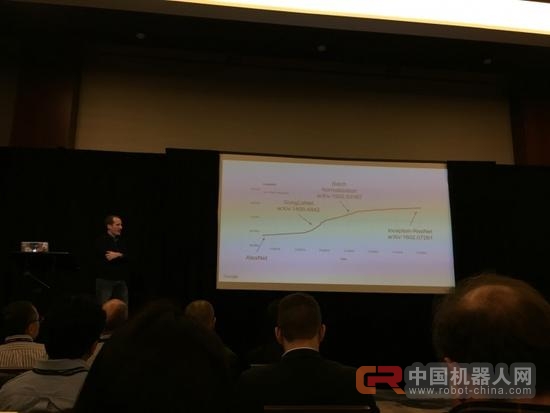
The machine grabs a specific object and it is possible to follow it. Grabbing an unknown object cannot solve it. (Vanhoucke broadcasts a robot wrestling highlights video, saying that in the past he thought these robots were wrestling and laughing, but after he started doing robotics projects, he couldn't laugh at this picture anymore. It's really hard to study robot crawling. , but he is very enjoyable.) The fewer image recognition techniques involved, the better the robustness of the machine and the ability to deal with more complex objects. When subsequent listeners asked about the database of objects, Vanhoucke said they (Google) did not want to create such a knowledge map.
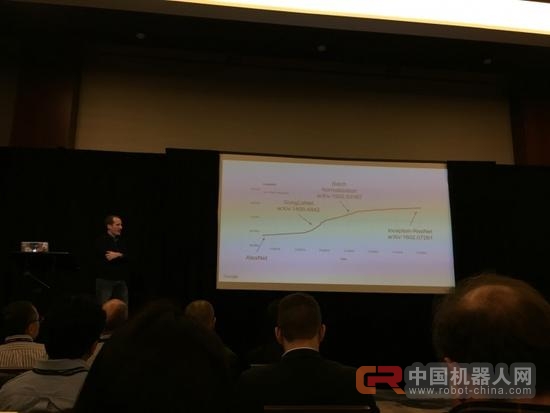
The introduction of reinforcement learning may be helpful for the study of robots, provided that there is a reference model that can generate a large number of samples. The final conclusion:
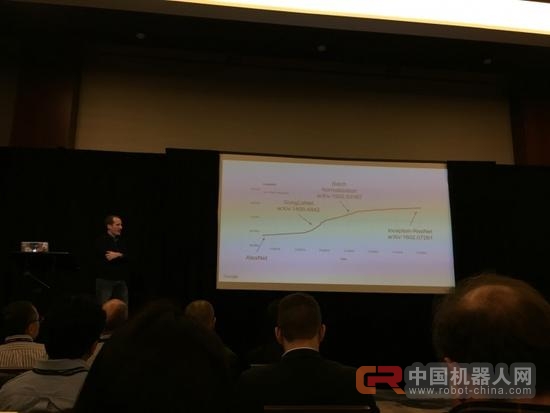
1. Robotics and machine learning are undergoing an interesting fusion; 2. For conventional robotics problems, there must be awareness of different answers; 3. It hits all the right difficult problems on the road to practical AI.
Tail Lamp
Tail Lamp,LED Tail Lamp,LED Combination Tail Lamp
Haiyu Automotive Lamp Co., Ltd. , http://www.nbautomotivelamp.com
没有评论:
发表评论